Chatbot Analytics: The Most Essential Metrics to Track in 2025
Chatbot analytics involves the systematic collection, analysis and interpretation of data generated by chatbots. Get familiar with the most essential metrics you must track in 2025.
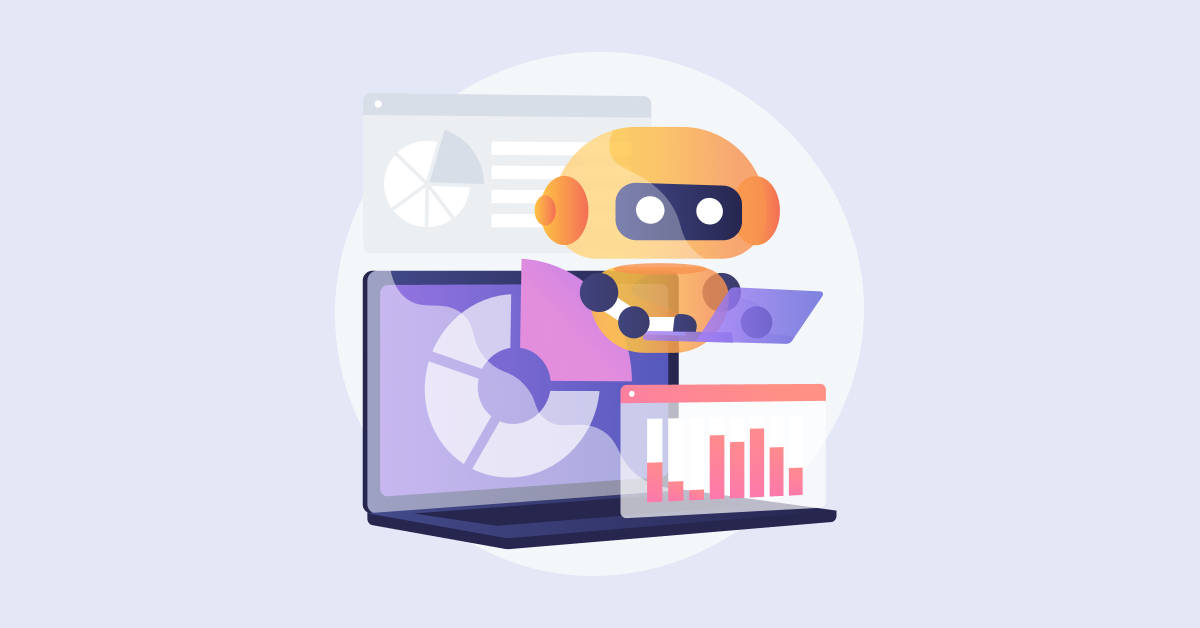
Chatbot analytics involves the systematic collection, analysis and interpretation of data generated by chatbots. Get familiar with the most essential metrics you must track in 2025.
One of the most innovative solutions that have gained immense popularity in recent years is the use of chatbots. But how can businesses ensure their chatbots are performing effectively? The answer is chatbot analytics!
Chatbots have quickly become a mainstay in customer service, offering a convenient and efficient way for businesses to interact with their customers. Chatbots will become a quarter of businesses’ primary customer service channels by 2027. Businesses can only unlock their true potential and provide the best user experience when they constantly gather insights about how their chatbot platform is performing.
The AI chatbot analytics allow businesses to analyze and understand the interactions with their customers. Businesses can make informed decisions to improve their chatbot’s performance by gathering data on user behavior, chatbot effectiveness and customer satisfaction. Let us discuss key metrics to track for optimal results.
Chatbot analytics refers to the systematic collection, measurement and analysis of data generated through interactions between chatbots and users. The field encompasses everything from basic usage metrics to sophisticated conversation pattern analysis, helping organizations understand how effectively their chatbots serve users and where improvements can be made.
The importance of chatbot analytics cannot be overstated in the modern industries. As businesses increasingly rely on automated conversation interfaces, understanding their performance becomes crucial for success. These analytics provide insights into user satisfaction, conversation flow efficiency and potential bottlenecks in the user experience.
Key principles:
Let us go through the importance of chatbot analytics to understand how harnessing the data can drive customer satisfaction.
1. Understanding user behavior: Chatbot analytics allows businesses to gain deep insights into behavior patterns. Companies can refine their chatbots to better meet customer requirements by analyzing data such as frequently asked questions, conversation lengths or specific user needs. The understanding empowers businesses to personalize the user experience, deliver targeted content and provide enhanced customer service.
2. Identifying performance issues: Chatbots are not infallible and there may be instances where they fail to provide accurate or satisfactory responses. Bot analytics enables businesses to identify performance issues such as incorrect or irrelevant answers, failed queries or user frustration points. The data can then be used to improve the chatbot’s responses, ensure a seamless user experience and minimize user frustration.
3. Improving conversion rates: Businesses can identify customer pain points and areas where potential customers may abandon interactions. The data-driven analysis allows companies to optimize their chatbots to guide active users through the sales funnel more effectively. Providing relevant information and engaging users proactively allows chatbot analytics to significantly improve conversion rates.
4. Streamlining workflows: Chatbots can handle a substantial volume of customer inquiries, freeing up human resources to focus on more complex or high-priority tasks. Businesses can identify frequently asked questions or common issues and develop automated responses. Keeping an eye on AI chatbot analytics streamlines workflows, reduces response times and allows businesses to operate more efficiently.
5. Personalized customer experiences: Chatbot analytics dashboard provides businesses with valuable data about individual customers, allowing for personalized experiences. Analyzing user interactions helps businesses to tailor chatbot’s responses to address specific customer needs or preferences. Personalization enhances customer satisfaction, builds brand loyalty and eventually leads to higher customer retention rates.
Below are the most essential metrics you need to track to gauge your chatbot’s effectiveness, drive engagement and deliver value to your organization.
Gaining insights into AI chatbot analytics and user metrics can help businesses identify areas for improvement. Let’s explore the importance of chatbot analytics and delve into the key user metrics you should be tracking to revolutionize the chatbot strategy.
When it comes to analytics of chatbots, one of the most important metrics to consider is the total number of users. 1.4 billion people is the count of the users who are open to using chatbots. Understanding the total number of users is crucial in optimizing and improving the overall performance of the chatbot. It helps in identifying patterns and trends, such as peak usage times or user drop-off points, which can be used to improve the chatbot’s user experience.
One use case for such chatbot metrics is in customer support. A high total number of users indicates that the chatbot is serving its purpose effectively, reducing the burden on customer support agents. A low total number of users might suggest that the chatbot needs improvement or more visibility.
Best practices:
Understanding user metrics is crucial for improving performance and enhancing customer experience. One of the key chatbot metrics that stands out is the number of new users. A significant increase in new users indicates that the chatbot is attracting attention and generating interest among potential customers. A declining number of new users might suggest the need for adjustments or improvements in your chatbot strategy.
A common use case for tracking the number of new users is when a company launches a new product or service. Businesses can effectively engage with potential customers and drive them towards purchasing by implementing a chatbot. Monitoring it allows companies to evaluate the success of their chatbot in attracting and converting new customers.
Best practices:
Chat volume is a crucial aspect of chatbot analytics that provides insights into the number of chats or conversations that take place between users and the chatbot within a specific period. It is a user metric that helps businesses understand the level of engagement and interaction with their chatbot. A high chat volume indicates the users are finding value in the chatbot and are using it frequently. A low chat volume may indicate that the chatbot is not meeting user expectations or that users are not yet aware of its existence.
One use case for chat volume is to assess the impact of chatbot enhancements or updates. Comparing chat volume before and after implementing changes allows businesses to measure the effectiveness of the modifications. If the chat volume increases after adding new features or improving the chatbot’s responses, it suggests the users are responding positively to the changes.
Best practices:
Goal completion rate (GCR) is a crucial metric in chatbot analytics that measures the percentage of users who complete a desired goal or task within the chatbot interaction. A high GCR indicates a well-designed and user-friendly chatbot that successfully assists users in achieving their goals. A low GCR highlights potential issues and bottlenecks in the chatbot’s flow or functionality.
One common use case of GCR in AI chatbot analytics is e-commerce. They are frequently used in online retail to guide users through the purchasing process. The GCR metric helps to track the users who completed a purchase or found the desired product, providing valuable insights into the chatbot’s impact on sales and customer satisfaction.
Best practice:
The number of bot sessions initiated holds significance as it indicates the level of user engagement with the chatbot. A higher number suggests that the chatbot is successfully attracting and retaining users, while a lower number may indicate a need for improvement. The metric helps in assessing the effectiveness of the chatbot in initiating conversation and driving user interactions.
One specific use case for tracking the number of bot sessions initiated is in customer support. Chatbots can be integrated into customer support platforms to handle common queries and provide assistance. Monitoring such bot metrics enables businesses to gauge the success of their chatbot in handling customer inquiries and resolving issues without the need for human intervention.
Best practices:
Bounce rate refers to the percentage of users who leave a chatbot without engaging in any further interaction. A high bounce rate is an indication that users are leaving the chatbot without receiving the information or assistance they were seeking. A high bounce rate suggests that the chatbot is not meeting user expectations or failing to provide the desired information. This information is valuable as it helps businesses identify areas for improvement and optimize the chatbot’s performance.
A common use case for bounce rate in the chatbot analytics metrics is in customer support. If a customer repeatedly fails to find the answers they need and consistently bounces from the chatbot, it indicates a significant flaw in the chatbot’s knowledge base or user experience. Businesses can identify the specific queries that result in high bounce rates and work on improving the chatbot’s responses or guiding users more effectively.
Best practices:
Let’s explore the importance of chatbot analytics and highlight the conversation metrics that businesses should pay attention to.
Conversation duration refers to the length of time a customer spends interacting with a chatbot. It provides insights into how long customers engage with the chatbot, indicating their level of interest and satisfaction. A longer conversation duration indicates that the chatbot is successfully meeting customer needs. The shorter durations may signal that customers are not finding the information or solutions they require.
One use case for conversation duration in chatbot analytics is identifying bottlenecks or areas where customers frequently drop off. Businesses can pinpoint where customers lose interest or face difficulties by analyzing conversation duration at different stages of the chatbot interaction. It allows for targeted improvements to enhance the chatbot’s effectiveness and user experience.
Best practices:
The interaction rate is a measure of how often users engage with a chatbot. It is calculated by dividing the total number of interactions with the chatbot by the total number of users. It is an important chatbot analytics metric as it provides insights into the level of user engagement and the chatbot’s effectiveness in fulfilling its intended purpose. A high interaction rate indicates that users find the chatbot helpful and engaging.
One common use case for the interaction rate is in customer support. It helps businesses identify areas where their chatbots excel in assisting and where they may need to improve. If the interaction rate is low during certain stages of a conversation, it may indicate the chatbot is not providing the desired information effectively.
Best practices:
Fallback Rate (FBR) is one of the top chatbot metrics that measures the percentage of conversations where the chatbot was unable to provide a satisfactory response and had to fall back to a human operator or default response. High FBR signifies potential gaps in the chatbot’s knowledge base or understanding of user queries, leading to frustrated and unsatisfied users. Monitoring FBR allows businesses to identify areas for improvement and refine their chatbot’s responses.
One prominent use case for analyzing FBR is in customer support services. Chatbots are often deployed to handle initial customer queries and provide immediate assistance. High FBR indicates that customers are not getting the answers they need, resulting in increased customer dissatisfaction. Monitoring the FBR consistently ensures that companies can identify common fallback triggers and train their chatbots to handle such scenarios effectively.
Best practices:
Assessing the satisfaction metrics allows businesses to optimize their chatbot’s performance and ensure the customers are not only satisfied but also delighted with the support they receive. Let’s explore them to understand how businesses can assess customer satisfaction when it comes to chatbot interactions:
Retention rate is also known as customer satisfaction metrics. It is a crucial aspect of the important chatbot analytics. Brands can measure the percentage of customers who continue to engage with their chatbot over a time frame. The metric provides valuable insights into how well the chatbot can retain customers and keep them satisfied. If they are optimized for retention, chatbots can generate about 20% repeat users.
One key use case for retention rate is in evaluating the success of chatbot updates and improvements. Keeping a check on the retention rate before and after implementing changes allows businesses to determine if the updates have effectively improved customer satisfaction.
Best practices:
The satisfaction score is typically given on a scale of 1 to 5, with 5 being the highest level of satisfaction. It takes into account factors such as ease of use, helpfulness and overall experience with the chatbot. A high satisfaction score indicates that the chatbot is effectively meeting customer needs, improving customer loyalty and increasing the likelihood of repeat business. A low satisfaction score highlights areas for improvement and the potential for customer dissatisfaction.
One common use case for the satisfaction score is in measuring the performance of chatbot enhancements or updates. Businesses should regularly compare their satisfaction scores to businesses. It helps them determine if the strategies they are implementing are positively impacting customer experience or if further improvements are still needed.
Best practices:
Businesses need to understand the importance of chatbot analytics to truly leverage the power of chatbots in marketing and drive commercial success. Let’s explore the essential marketing and commercial metrics that businesses should focus on when it comes to chatbot metrics.
Return on Investment (ROI) is a crucial metric for any business but when it comes to chatbot analytics, it plays an even more significant role in evaluating the success and effectiveness of a chatbot marketing campaign. ROI measures the profitability or cost-effectiveness of an investment relative to the amount of money invested. It helps measure the return on investment for marketing and commercial efforts related to chatbots.
One use case for tracking ROI in AI chatbot analytics is measuring the impact of chatbot-driven lead-generation campaigns. Businesses can determine the conversion rate, cost per lead and overall profitability of their chatbot-generated leads by analyzing ROI. It helps in optimizing marketing efforts, improving customer targeting and increasing the return on investment from chatbot-driven lead generation.
Best practices:
Leads generated is an analytical metric that measures the number of potential customers who have shown interest in a product or service through a chatbot interaction. It provides valuable insights into customer behavior and allows businesses to gauge the effectiveness of their chatbot marketing strategies.
One compelling use case for leads generated in analytics for chatbots is lead nurturing. Companies can collect contact information from potential customers and use it to nurture leads through targeted email campaigns or personalized follow-ups. It can help identify areas of improvement in the chatbot’s design and performance, allowing for iterative enhancements that result in increased lead generation.
Best practices:
Following are the key features of chatbot analytics tools that can transform data into growth opportunities and enable your business to stay ahead of the competition.
1. Easy to use: One of the key features of chatbot analytics tools is their user-friendly interface. Such tools are designed to provide businesses with easy access to data and insights, even for the ones who may not have a technical background. The chatbot analytics dashboard should be intuitive and provide a clear overview of chatbot performance.
2. Customization: Businesses have diverse needs and goals when it comes to chatbot analytics. The top chatbot analytics tools should allow customization, enabling businesses to track specific metrics that align with their objectives. It could include tracking customer satisfaction ratings, conversation flow, conversion rates or any other metrics that are relevant to the business.
3. Multiple seats: Chatbot analytics tools should support multiple users and provide different access levels. The feature is particularly useful for businesses that have multiple teams or departments working on chatbot management. Having multiple seats allows the team members to collaborate and access the analytics data they need to optimize chatbot performance.
4. Team performance tracking: Effective chatbot analytics tools should not only track individual user interactions but also provide insights into team performance. It includes metrics such as response time, resolution rate and overall customer satisfaction across the team. Businesses can identify areas where additional training or resources may be needed to enhance their chatbot team’s performance.
5. Goal tracking: Chatbot analytics tools should allow businesses to set and track their specific goals. Whether it’s improving customer engagement, increasing sales conversion or enhancing customer satisfaction, the tool should provide visualizations and reports to monitor progress towards the goals. It enables businesses to make data-driven decisions and optimize their chatbot strategy accordingly.
6. Mobile display: All of the industries have become mobile-centric in one way or another. It has become essential for chatbot analytics tools to have mobile display capabilities. Businesses can access their analytics data on-the-go, from their smartphones or tablets. Mobile display ensures that businesses can stay informed and make real-time decisions, even when they are away from their desks.
7. Sentiment analysis: Understanding customer sentiment and emotions is crucial for effective chatbot interactions. Chatbot analytics tools with sentiment analysis capabilities can analyze the language and tone used by customers during their conversations. It allows businesses to gain insights into customer satisfaction levels, identify pain points and tailor their chatbot responses accordingly.
Chatbots have become an integral part of the customer service strategy for many businesses. They offer efficient ways to communicate with customers and provide instant support. If businesses want to enhance their chatbot strategy truly, they need to leverage advanced analytics.
Analyzing the data collected from chatbot interactions allows you to gain valuable insights into customer preferences, behaviors and pain points. The information can be used to refine the chatbot’s responses, personalize the customer experience and identify areas for improvement. The advanced analytics empower businesses to optimize their chatbot strategy to drive better engagement, satisfaction and business success. Make sure you don’t overlook the power of analytics in maximizing the potential of the chatbot.
The AI chatbot analytics provide valuable insights into user behavior, preferences and interactions. Analyzing data such as user engagement, response time and conversion rates allows businesses to optimize their chatbot performance. They can easily tailor their responses to meet customer needs. The AI bot analytics allows companies to make data-driven decisions, improve customer satisfaction and enhance their overall digital marketing strategies.
Analyzing a chatbot involves assessing its performance in terms of user experience, accuracy of responses and effectiveness in achieving its intended purpose. It can be done through reviewing chat logs, conducting user surveys, tracking user interactions and implementing A/B testing to optimize the chatbot’s performance. It is essential to continuously monitor and analyze the chatbot’s data to make data-driven improvements.
Developers use custom chatbot analytics data to improve chatbot interactions by analyzing user engagement, conversation flows and response effectiveness. The data helps identify areas of improvement and allows developers to make necessary adjustments to optimize the chatbot’s performance. They can fine-tune the chatbot’s responses, add new features and enhance its overall functionality based on insights gathered from the analytics data.
Analysis of chatbot analytics enables businesses to identify areas of improvement in their chatbot’s performance and make adjustments to enhance customer experiences. Let’s say if the analytics reveal the users are frequently dropping off during a specific stage of the conversation, businesses can revise the chatbot’s responses to address common user concerns.
Market better, sell faster and support smarter with Veemo’s Conversation Customer Engagement suite of products.
Unify all your customer data in one platform to deliver contextual responses. Get a 360 degree view of the customer lifecycle without switching tools.
Connect with the tools you love to reduce manual activities and sync your business workflows for a seamless experience.
Grow Customer Relationships and stronger team collaboration with our range of products across the Conversational Engagement Suite.